Research & Academic
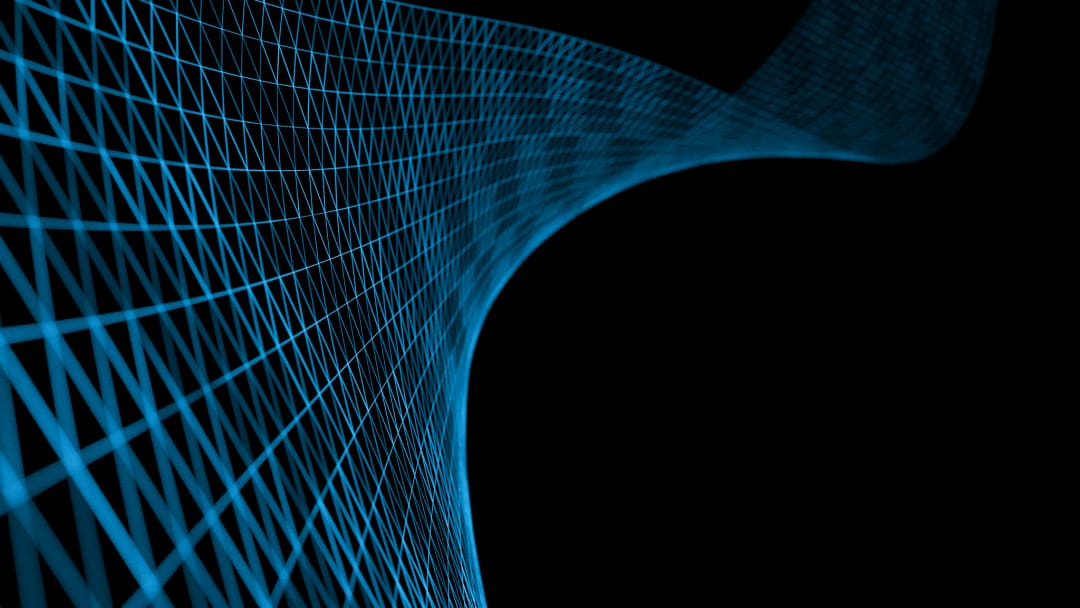
Application clustering: machine learning approach to datacenter network abstraction
Well defined and strict communication policies between applications reduce attack surface and restrict possible lateral movement across the network. However, companies often have very limited information about the applications running in their environments – where the applications are deployed, what their boundaries are and how they interact with each other. We introduce a novel machine learning approach that uses network flows to generate application-level representation of public and private cloud networks. This will greatly simplify the journey to a micro-segmented network.
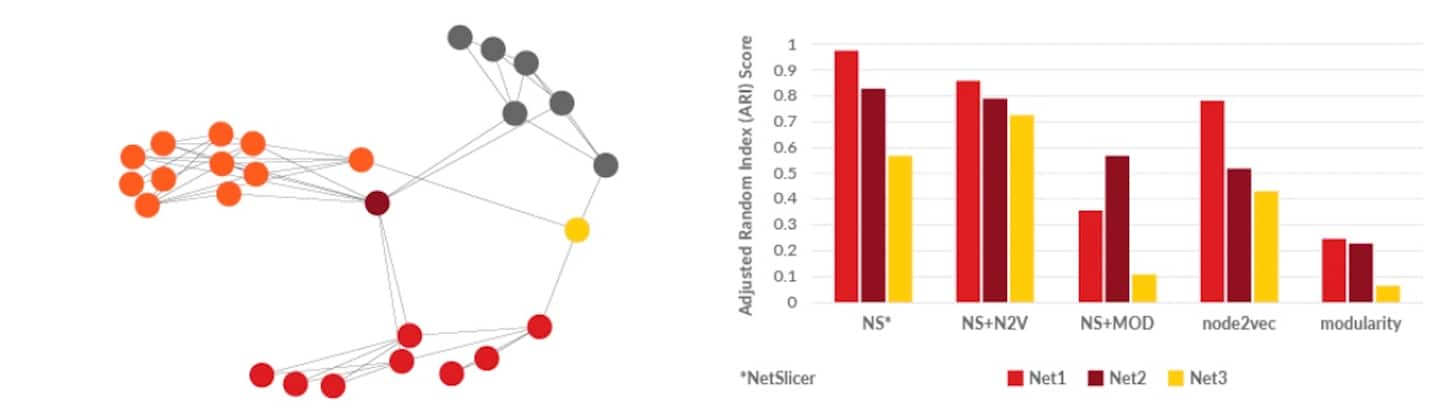
Teleportation attacks: vulnerability in the SDN architecture
Software-defined networking (SDN) is considered a promising new paradigm, enabling more reliable and formally verifiable communication networks. Our research shows that what forms the basis for SDN – the separation of the control plane from the data plane – is vulnerable to an attack we are referring to as teleportation. An attacker, e.g., a malicious switch or a host, can transmit (“teleport”) information via the control plane, bypassing critical network functions in the data plane (e.g., a firewall), and violate security policies as well as logical and even physical separations.
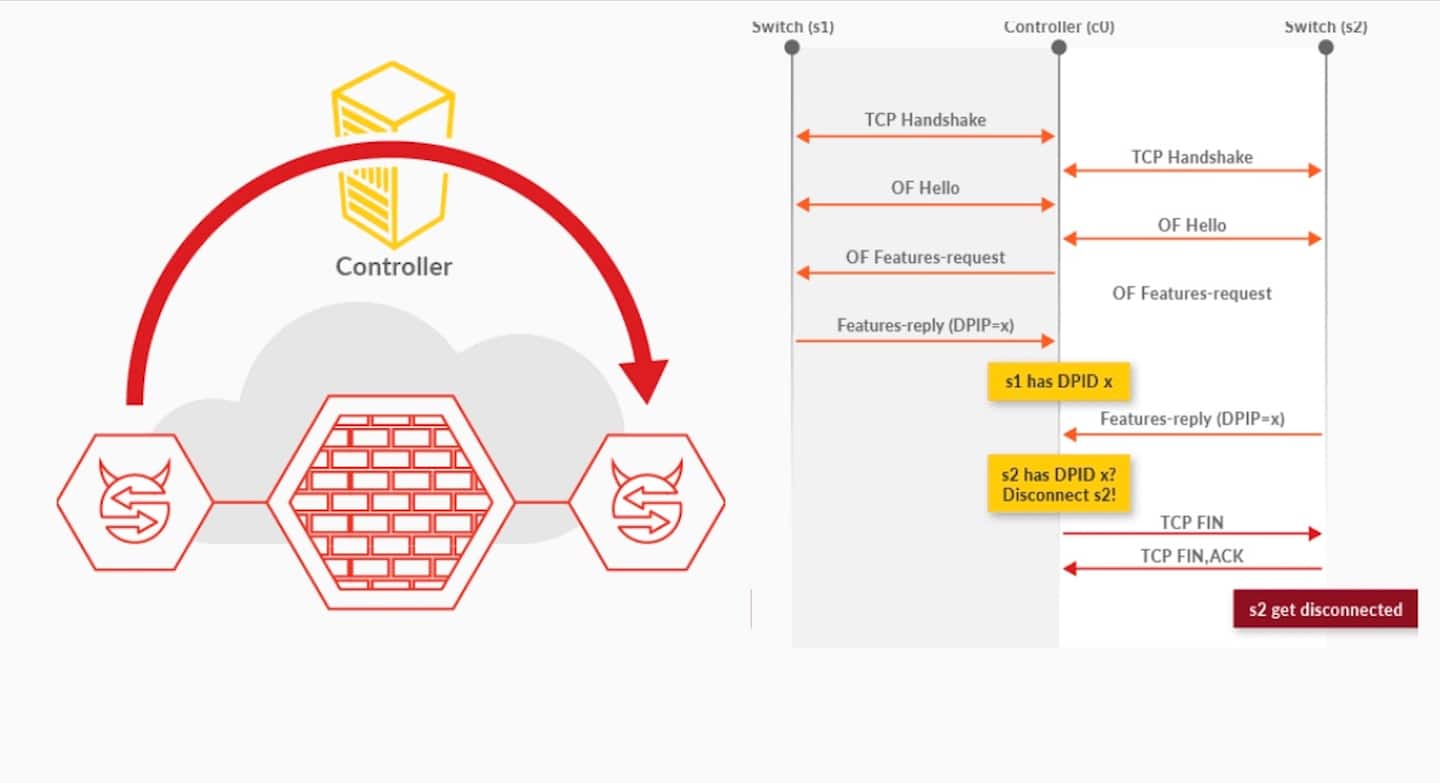
SDN robustness: self stabilization and fault tolerance
Software-defined network (SDN) architectures raise the question of how to deal with situations where the round trip via the control plane is not fast enough or not possible. In order to provide a high availability, connectivity, and robustness, dependable SDNs must support basic functionality also in the data plane. In our research we show how to extend SDNs with functionality for self-stabilizing in-band control and failover routing in the presence of link failures.
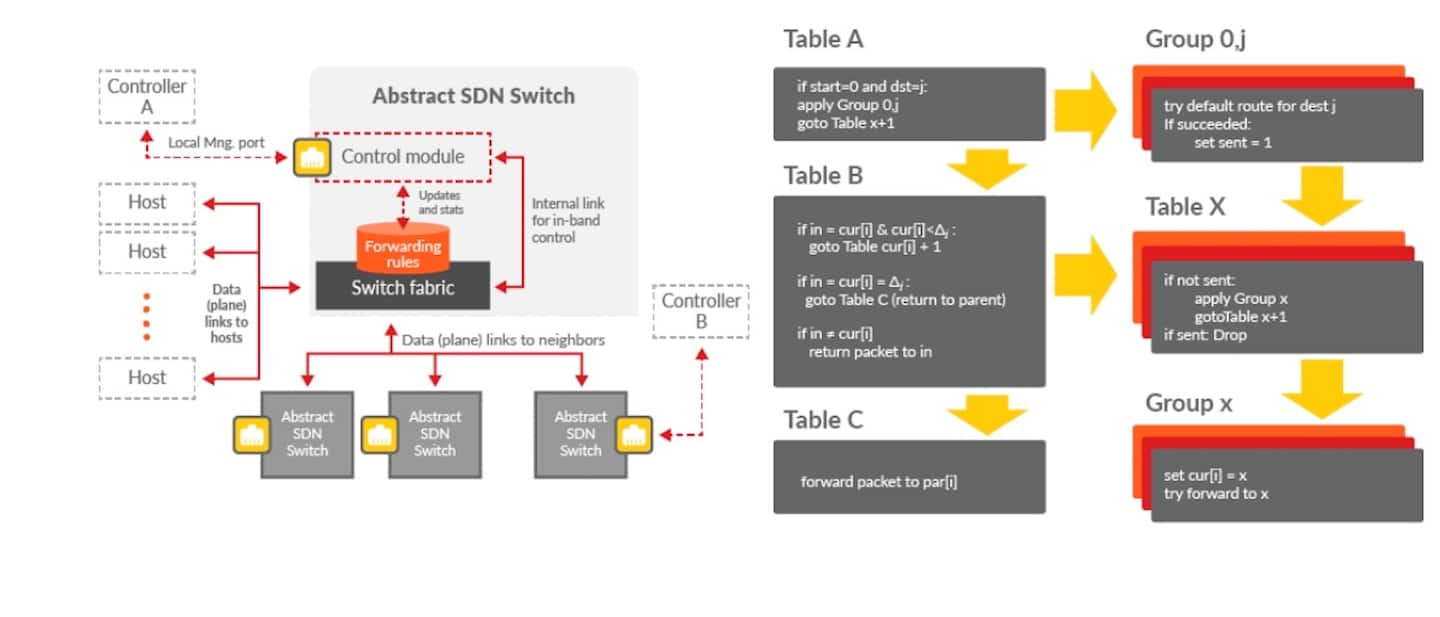